Machine Learning Against Fraud in Canadian iGaming
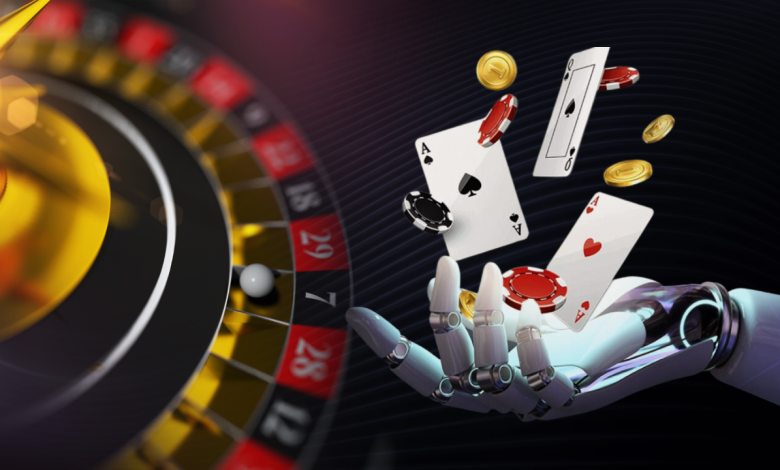
When it comes to online gambling, Canadian iGaming companies face a constant battle against fraud, money laundering, and other illicit activities that threaten the integrity of their platforms and the trust of their players. To combat these challenges, many operators are turning to advanced machine learning algorithms to enhance their fraud detection capabilities while ensuring compliance with regulatory requirements. In this article, we’ll explain how gambling websites Canada leverage machine learning techniques to safeguard their platforms and maintain player trust.
Introduction to Machine Learning in iGaming
Machine learning, a subset of artificial intelligence, empowers computers to learn from data and make predictions or decisions without being explicitly programmed. In the context of iGaming, machine learning algorithms analyze vast amounts of data generated by player interactions, transactions, and gameplay to identify patterns indicative of fraudulent behavior. By continuously learning from new data, these algorithms adapt and evolve to stay ahead of emerging threats.
Techniques Utilized in Fraud Detection
- Anomaly Detection: One of the primary techniques employed by Canadian iGaming companies is anomaly detection, which involves identifying deviations from normal behavior that may indicate fraudulent activity. For example, if a player suddenly starts making unusually large deposits or exhibits erratic betting patterns, it could be a red flag for potential fraud.
- Predictive Modeling: Predictive modeling techniques, such as logistic regression and decision trees, are used to forecast the likelihood of fraudulent behavior based on historical data and known risk factors. By analyzing variables such as player demographics, transaction history, and gameplay patterns, these models can identify high-risk individuals or transactions deserving of further scrutiny.
- Natural Language Processing (NLP): In cases where fraud attempts involve textual data, such as chat transcripts or customer support interactions, NLP techniques are employed to extract relevant information and detect suspicious patterns or keywords indicative of fraudulent behavior. For instance, NLP algorithms can analyze chat logs to identify players discussing collusion or match-fixing activities.
- Network Analysis: Network analysis techniques, such as social network analysis and graph theory, are utilized to identify connections and relationships between players or entities involved in fraudulent activities. By visualizing these networks and detecting unusual patterns of interaction, Canadian iGaming companies can uncover complex fraud schemes spanning multiple accounts or platforms.
Real-World Examples
Case Study 1: Detecting Collusion in Online Poker
In 2019, a Canadian iGaming operator detected suspicious activity on its online poker platform involving multiple players consistently winning large sums of money in high-stakes games. Using machine learning algorithms to analyze gameplay data and player interactions, the operator uncovered evidence of collusion, where players were secretly working together to gain an unfair advantage. By promptly identifying and banning the colluding players, the operator safeguarded the integrity of its platform and protected honest players from exploitation.
Case Study 2: Preventing Money Laundering in Sports Betting
In 2020, a leading Canadian sports betting platform implemented machine learning-based anti-money laundering (AML) measures to combat illicit activities such as match-fixing and money laundering. By analyzing betting patterns, transaction histories, and other relevant data, the platform’s algorithms flagged suspicious transactions indicative of potential money laundering schemes. Subsequent investigations led to the identification and reporting of suspicious activities to regulatory authorities, ensuring compliance with AML regulations and preserving the platform’s reputation for fair play.
Ensuring Regulatory Compliance and Player Trust
While machine learning algorithms play a crucial role in detecting and preventing fraud in Canadian iGaming, operators must also prioritize regulatory compliance and player trust. To achieve this, operators implement robust Know Your Customer (KYC) procedures to verify the identity of players and adhere to strict anti-money laundering (AML) regulations outlined by regulatory authorities. Additionally, transparent communication and proactive measures to address player concerns contribute to building and maintaining trust within the iGaming community.
Conclusion
In conclusion, the utilization of machine learning algorithms in Canadian iGaming represents a pivotal advancement in fraud detection and prevention. Through techniques such as anomaly detection, predictive modeling, natural language processing, and network analysis, iGaming companies are equipped to identify and mitigate risks posed by fraud, money laundering, and collusion. Case studies have demonstrated how Canadian iGaming operators successfully detected and addressed instances of collusion in online poker and prevented money laundering in sports betting through the implementation of machine learning-based anti-fraud measures.
To ensure continued success in combating fraud and maintaining player trust, it is imperative for Canadian iGaming companies to prioritize regulatory compliance, transparent communication, and proactive measures to address player concerns. By adhering to robust Know Your Customer (KYC) procedures, adhering to anti-money laundering (AML) regulations, and fostering open dialogue with players, operators can uphold the integrity of their platforms and foster a safe and enjoyable gaming environment.
FAQ
Q: How do machine learning algorithms detect fraud in iGaming?
A: Machine learning algorithms analyze vast amounts of data generated by player interactions, transactions, and gameplay to identify patterns indicative of fraudulent behavior, such as anomalies in betting patterns or suspicious account activity.
Q: What are some examples of fraud detection techniques used in Canadian iGaming?
A: Canadian iGaming companies utilize a variety of techniques, including anomaly detection, predictive modeling, natural language processing, and network analysis, to detect and prevent fraud, money laundering, and collusion on their platforms.
Q: How do iGaming operators ensure compliance with regulatory requirements?
A: iGaming operators adhere to strict Know Your Customer (KYC) procedures to verify the identity of players and comply with anti-money laundering (AML) regulations outlined by regulatory authorities. Additionally, operators implement robust fraud detection measures and cooperate with regulatory agencies to ensure compliance.
Q: What measures can players take to protect themselves from fraud in iGaming?
A: Players can protect themselves by choosing reputable iGaming operators licensed by regulatory authorities, ensuring the security of their accounts with strong passwords and two-factor authentication, and being vigilant for any suspicious activity or communications.
Q: How does machine learning contribute to responsible gambling practices in iGaming?
A: Machine learning algorithms can analyze player behavior and identify signs of problem gambling, allowing operators to intervene and provide support to at-risk players. Additionally, machine learning can personalize responsible gambling messages and interventions based on individual player profiles.